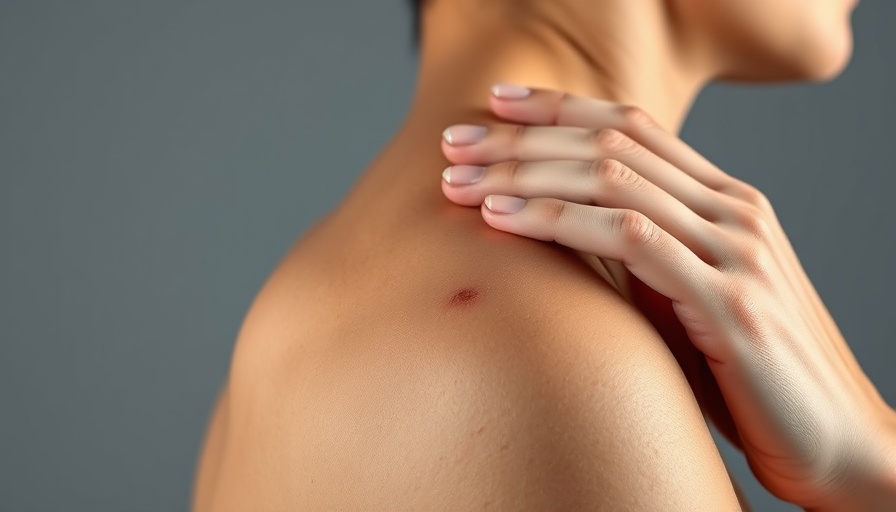
Revolutionizing Skin Diagnostics: A Deep Dive Into AI Innovations
In a significant breakthrough for dermatological diagnostics, a new study has unveiled a deep learning model capable of accurately differentiating keloids from malignant skin lesions using non-dermatoscopic clinical images. The VGG16 convolutional neural network (CNN) achieved impressive metrics including an accuracy of 0.985 and precision of 1.0, boasting an area under the receiver operating characteristic curve (AUC) of 0.996. This innovation presents a profound opportunity for enhancing diagnostic accuracy in settings lacking dermatology specialists or dermatoscopic imaging.
Understanding Keloids and Their Diagnostic Challenges
Keloids are abnormal growths of scar tissue that can occur at the site of skin injuries, often mistaken for malignant conditions due to shared characteristics like uncontrolled growth and high recurrence rates. This similarity raises crucial concerns; incorrect diagnoses can lead to postponed treatment, resulting in adverse outcomes for patients. The findings from this recent study underscore the necessity for accurate diagnostic tools in distinguishing these common yet problematic skin lesions.
AI Performance: Beyond Traditional Models
VGG16 outshined eight other pretrained CNNs in two out of three key classification tasks after fine-tuning, showcasing the potential advantages of tailored AI models in dermatology. This is particularly relevant as dermatologic cases often present a challenge for clinicians who might not have immediate access to specialized tools. Exploring how neural networks outperform traditional classification approaches not only emphasizes the efficacy of AI in medical fields but also highlights the importance of continuous development in training and data acquisition to improve model reliability.
The Importance of Diverse Training Data
While VGG16 demonstrated superior classification performance, the study also revealed challenges. The reliance on image quality and limited datasets could affect the model's overall generalizability. Ensuring a diverse array of training data, integrating clinical and imaging features into the training process, and broadening the range of skin types represented could further enhance the model's capabilities. As technology continues to evolve, it becomes increasingly essential to address these gaps to maximize the benefits of AI advancements in patient care.
Future Implications for Dermatology Practice
As the healthcare industry increasingly embraces AI, the future of dermatological diagnostics looks promising. Integrating AI tools like VGG16 into everyday practice could alleviate some of the strain caused by physician shortages and improve patient outcomes through timely and accurate diagnoses. Furthermore, empowering non-specialist clinicians with AI-backed tools might democratize access to quality care, particularly in rural or underserved areas where dermatological expertise is scarce.
Conclusion: Stepping Into the Future
The potential for deep learning models like VGG16 represents a paradigm shift in the diagnostic capabilities of dermatology. With continued advancements in AI and machine learning, the dermatology field can enhance how patients are diagnosed and treated, ensuring that care is not only equitable but also effective. As these technologies mature, expect greater integration of AI diagnostics into comprehensive patient management strategies.
For those invested in skincare and dermatology innovation, understanding these emerging technologies can provide valuable insights into the future of medical care. Stay informed, as the fusion of technology and healthcare promises to reshape the landscape significantly.
Write A Comment